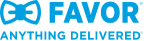
Austin-based delivery company Favor uses PredictHQ to accurately match supply to demand on a local level
So far, Favor has seen up to a 6% improvement in MAPE when incorporating features based on PredictHQ data.
Use Case
Demand Forecasting
Industry
Transportation
Products & Features
Learn more
Introducing Favor
Delivery companies are skyrocketing in popularity across the world with the top four U.S. food delivery companies enjoying a $3 billion collective revenue increase in six months in 2020. The impact of COVID-19 pandemic is not limited to 2020, as consumer habits have changed forever, especially the growing reliance on delivered food, drinks and more.
Favor, a rapid growing disrupter in Austin, Texas, is no exception. Favor offers anything delivered in under an hour and currently covers 200 cities across Texas. Orders are placed through its app or website and then couriers transport orders from stores to customers.
Riding the surging wave of demand for food-delivery, Favor’s biggest priorities are ensuring on-time deliveries, making sure customer experience is top-notch and accurate forecasting to reduce costs. They need to know more than “general demand is up.” The highest impact solution to their challenges, which are shared by delivery companies globally, is to always understand what is going on in each neighborhood they service. Paying attention to the local communities and what is impacting demand on a hyper-level means they can optimize their business and service.
Favor focused on building a strong data science team and methodologies from the start
Kevin Johnson, Head of Data Science for Favor joined in January 2020 and was tasked with building the data science organization from the ground up. One of his early goals was to bring in any external data sources to drive efficiency and great service, but also to lay the data foundation to rapidly scalable models to unlock more use cases later down the line. Core to both was understanding what drives demand.
When vetting new sources of external data, they looked at what could help them achieve more accurate, unbiased, forecasts. Underestimating demand for Favor means a limited number of drivers, missed delivery SLAs, an increase in customer complaints, more order cancellations or refund requests and more. Overestimating demand leads to higher driver costs and incentives and idle and frustrated drivers.
Favor’s forecasting models work with both long- and short-term forecasting horizons. Certain levers can be pulled two weeks in advance compared to the most impactful levers on the day of. Both can be high impact, so the data science team is always working to improve their forecast KPIs.
One of the first areas they investigated was events. They knew anecdotally events, both small scale and large—such as concerts and fun runs—impact food-delivery demand, but they needed to find a trusted source of intelligent external data which could make their platform more real-world aware to adapt to demand changes in the neighborhoods and cities they service across Texas.
Favor partners with PredictHQ to access 8 event categories across the state of Texas
Johnson discovered PredictHQ’s offering in early 2020. His criteria was simple: can PredictHQ give us useful data around events that will ultimately improve our forecasts? After a successful pilot where they incorporated event data into models for specific cities against baseline versions of the models, they quickly saw value.
PredictHQ’s easy-to-use Event API gave Favor direct access to real-world event data, verified from billions of data points every day. Every event is cleansed, filtered, enriched, verified, and then ranked based on predicted impact with proprietary ranking technology. Importantly, every event was enriched with a unique predicted attendance model that factored in pandemic restrictions, and all event details were constantly reverified. PredictHQ is enabling the Favor team to gain additional context at the local level for every city they service.
Favor was also drawn towards the unique categories captured in the dataset. PredictHQ has 19 different event categories that span from a sports game, to a college graduation, to a severe weather event such as hurricane warnings. Accessing insight into such a diverse set of events has unlocked endless possibilities for the team. They feel like they are just getting started.
"The granularity and diversity of PredictHQ’s event categories have helped us get the full story, enabling us to identify and understand demand within individual neighborhoods at scale,” Johnson says.
PredictHQ’s team played a hands-on role with Johnson to identify which categories impacted their areas of focus specifically and to identify specific model features, like aggregations that have since been automated with PredictHQ’s Features API.
Barel Alcantara is a Senior Data Engineer at Favor. Alongside Johnson, he was tasked with incorporating PredictHQ data into Favor’s systems and models. He has found the API to be thorough and flexible, with things like a search capability within the API or the ability to reference historical data. For context, another API the Favor team was working with around weather data had a different schema for historical data, which required more development and resources.
Alcantara is leading a project to overhaul how they ingest external data to ensure they are working with the most up-to-date data. This reduces models latency and maintains the most competitive tech stack. Favor was ingesting PredictHQ data through multiple AWS Lambda functions when they first started working with the team - this was proving to be unstable at times. They have now refactored and transitioned to AWS Glue—using Spark as their ingestion engine for large scale and distributed data processing—this has made all the difference. They are able to call the API in parallel for each different Favor neighborhood within a single job, and if there are any errors, they are able to pinpoint and quickly diagnose. The Favor team works with roughly 90 days worth of data from the API every day, 7 days historical and 3 months forward-looking. Effective data ingestion and processing are critical to building effective machine learning models supporting their business.
Alcantara wanted to share with other data engineers/scientists looking to incorporate PredictHQ’s event data, “You should absolutely leverage PredictHQ’s documentation, data exporter, and notebooks. The notebooks in particular do a great job showcasing common ways that the data is used, such as knowing nuances for specific categories, and how to filter out the noise. This level of documentation is unique and very useful for a data company.”
Results
So far, Favor has seen a 5 - 6% improvement in MAPE while the MAE improvement was more moderate when incorporating features based on PredictHQ data. The improvement was largely seen in smaller markets where events likely cause a bigger fluctuation to their business. Johnson shares, “We're looking forward to continuing to use PredictHQ data as inputs for additional forecasting efforts and other ML applications."
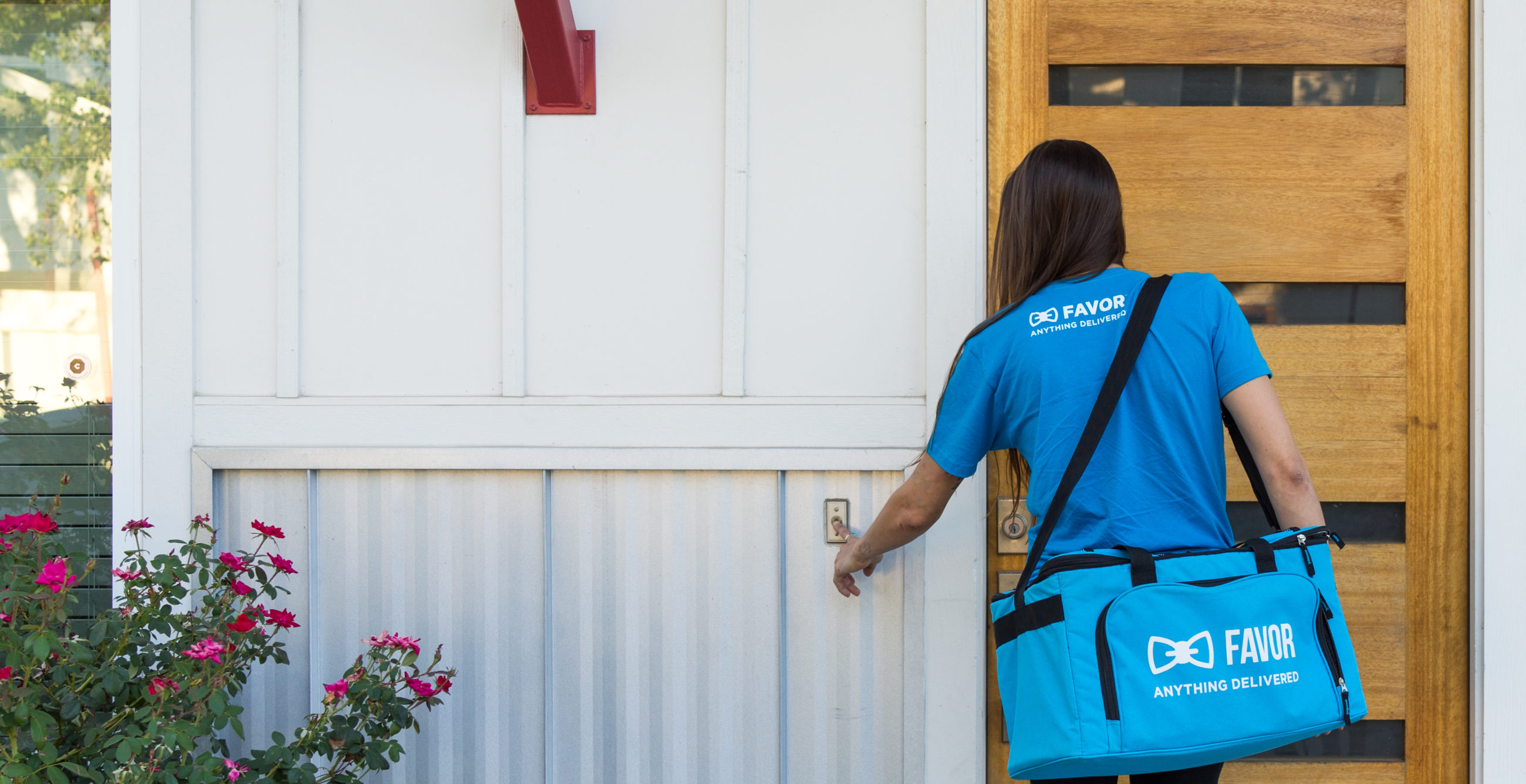
So far, Favor has seen up to a 6% improvement in MAPE while the MAE improvement was more moderate when incorporating features based on PredictHQ data.
Favor is just scratching the surface with the possibilities for event data. Along with constantly testing new features and categories to further improve their success, Johnson has been an active member of PredictHQ’s User Advisory Board, which allows him and the Favor team to help test and build future features and products. The two newest features that the team is excited about is the Features API and Demand Impact Pattern -- the latter one will allow them to understand the full picture of demand for severe weather events, including the days leading up to and following a severe weather event.
Johnson’s final piece of advice is, “Don’t try to reinvent the wheel. Take the lessons from companies who have gone through bringing on external datasets and directly from PredictHQ.”