Forecast grade data: how to assess data providers to ensure quality
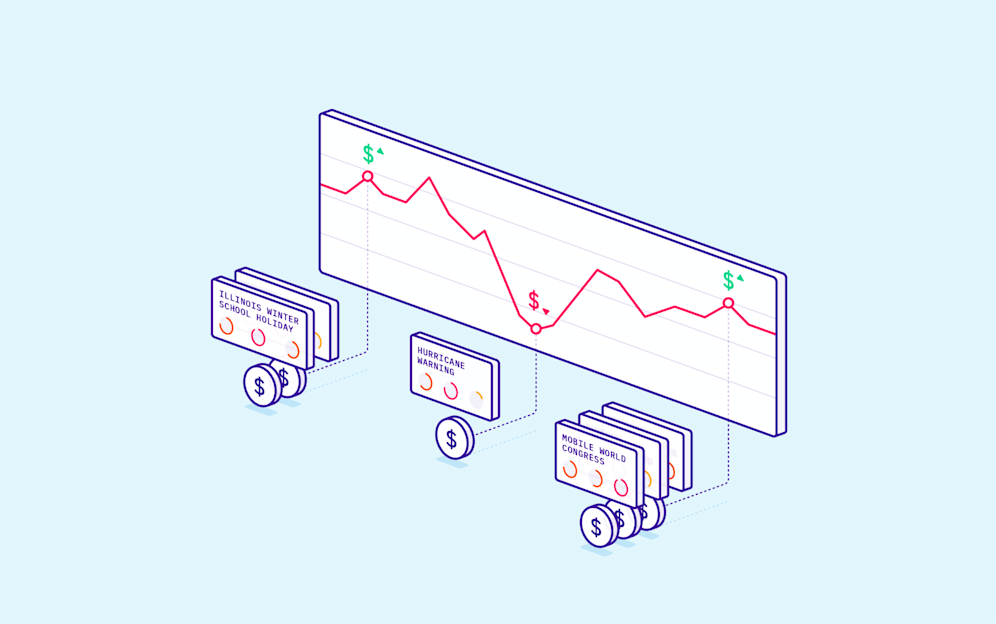
Garbage in equals garbage out. It’s true about many things in life and it’s staggeringly true for data science. With more companies turning to data-driven forecasting to make sense of these chaotic period, it’s time to talk about why forecast grade data is the only data you should use to inform your decision making.
As the world readies for the next normal with COVID-19, the businesses that are finding their feet fastest are those that are data-driven and doubling down on these capabilities. McKinsey puts it well in a recent report: “Chief data officers in every industry will play a critical role in crisis response and the next normal that follows. In today’s high-stakes environment, where misinformation proliferates and organizations must make decisions at a rapid pace, there’s arguably never been such an imperative for CDOs to provide organizations with timely and accurate data.”
Timely and accurate data sounds simple. Yet it’s estimated data scientists lose 80% of their time finding and fixing data rather than creating models and value with it. This is because there are so many data quality issues that can break machine learning models. If you work in a data-driven organization, this is a problem you are either painfully aware of, or one you need to grapple with as quickly as possible.
Assessing quality with the six industry standard dimensions of quality
When assessing data, there are six types of quality to consider to assess a data source’s reliability and value for your business. These are:
Accuracy
Completeness
Uniqueness
Timeliness
Validity
Consistency
The exact metrics or merits of each element will vary depending on the kind of data but you must apply all six. It is critical to bring experienced data scientists into the assessment process as early as possible and empower them to drive a highly effective assessment criteria based on these six industry standards.
The criteria for weather data will be distinct from the criteria for exchange rate and currency data, which will be distinct again from consumer sentiment and movement data. In our case, PredictHQ ingests event data from hundreds of sources – some public and some proprietary. This has enabled us to devise and launch Quality Standards for Processing Demand Causal Factors – the standard for event and demand-impacting data. So the intricacies of assessing data and the complexities is a topic we know well.
Here are examples of the questions to ask around two of the industry standards above: accuracy and validity.
For accuracy, our top line questions when assessing data are:
Are the core details (location, time, date etc) accurate?
Is it accurately classified into its event type so it’s easy to find and understand? Ie a sports game or a public holiday.
Have our machine learning models accurately calculated the predicted attendance?
And critically in this COVID era, is the event’s state accurate? Ie is it active, postponed or cancelled.
For validity, especially when we’re considering adding a new source of data to our pipeline, our topline questions include:
What proportion of these events are spam events? How many are duplicate events? For many of our data sources, this is between 10 to 30% before our models find and delete these misleading events.
What percentage are virtual only? For example, PredictHQ has removed over 28,000 virtual events in 2020 that do not cause physical demand.
Are events that don’t cause people movement but can cause demand, such as closed-door sports events or Live TV events, clearly labelled?
Assessing value and if the data is fit-for-purpose within your organization
Once you have confirmed a new data source is high quality, the next step is to assess the value to your operations. Putting a new data source through its paces is a critical quality management step because it reveals:
If the format of the data can be used by your machine learning models or what kind of work it will need to before it can provide value
How relevant the data set is to your operations
The best way to use it to drive results for your business as quickly as possible
This requires substantial amounts of historical data, both your own and the providers. Historical data is crucial for understanding what impact a new data source has had and therefore will have. For forecasting, historical data enables you to find correlation as well as reduce your demand forecasting error rate by revealing causes for previously unexplained anomalies.
Extensive historical data is particularly important to prepare for the next normal because of the disruption of COVID-19. It is a mistake to throw out 2020’s data and rely on previous years, as the world won’t return to normal overnight, even with a successful vaccine. You need to be able to identify the connection and intelligence that a new data source provides across multiple years to get insight worth building forecasts on.
For PredictHQ, our customers start by correlating our data to their historical transactional data. This reveals which events impact their demand, both positively and negatively, so they can build far more accurate forecasts that mitigate the losses of decremental demand and seize the opportunity of demand driven by events.
Assessing scalability and ease of use is essential for forecast grade data
Once you’ve established a new data source is high quality and useful for your business, the final hurdle is if it can be used in a scalable way by your team.
Many of the characteristics that make data scalable and easy to use can be baked into the sub-questions of the six industry dimensions of quality. For example: standardization is a critical component of consistency. But we want to call this step out independently because it is particularly important at the moment as most companies are running with fewer team members than previously.
Having one uniform format is critical, especially when wrangling many different kinds of information. For example, PredictHQ tracks 19 categories of events, which vary from unscheduled severe weather events through to sports games to observances. Each of these have different impact, durations, locations and come from a range of sources, so require different enrichment.
Not only does data need to be easy for your models to ingest, but it must produce actionable output. For example, data that has ineffective de-duplication models will forecast false-positive demand, causing your team to prepare for a surge that does not occur, potentially wasting millions.
This is why intelligence layers built on top of verified data is critical for forecast grade data. The era of big data was exciting but frustrating, and out of the many lessons learned by business is the requirement for smart data, rather than simply endless reams of data your team will need to build models on to parse, prioritize and find value in.
While it can be tempting in these uncertain times to opt for DIY options, the damage this could do to your planning and team’s productivity will be radically more expensive than paying for the best data so your team can focus on forecasting. The Harvard Business Review sums it up well: “Companies need to avoid making shortsighted decisions about data infrastructure and human resources… In our post-stay-at-home reality, companies need to recognize that their existing predictive models, forecasts, and dashboards may all be unreliable, or even obsolete, and that their analytic tools need recalibrating.”
If you are assessing a demand intelligence offering for your forecasting models, our team is easy to contact to compare our forecast grade data with others. For more on how PredictHQ’s 1000+ machine learning models find and fix data quality issues in event data before events enter our API, click here. For how to make event data more reliable for forecasting, check out this article.